Sent Successfully.
Data Science
- Data Analytics in Automotive Industry
- Media Analytics
- Oil Prices and Stock Market Analysis using K-Means Clustering
- Travel Analytics
- Top 10 Python Libraries for Data Science
- Top 10 Data Mining Applications: A Complete Guide
- Applications of analytics in Construction Industry
- Manufacturing Analytics
- Machine Learning in the Airline Industry: The Next Step
- Applications of Data Analytics in the Education Sector
Artificial Intelligence
- Object Detection with Auto Annotation
- Object Detection
- What is TensorFlow? Harnessing the Power of Deep Learning
- Boltzmann Machines and Energy-Based Models: Unleashing the Power of Artificial Neural Networks
- Caffe Tutorial : Applications and Key Features
- Introduction to Deep Learning: Key Components and Future
- The Future of Deep Learning: Challenges and Opportunities
- What are Generative Models and Examples
- What is Recurrent Neural Network
- Variational Autoencoders Tutorial
Domain Analytics
- How Data-Driven Technology Can Transform The Financial institutions
- Sales Analytics
- Supply Chain Analytics: What It Is & Why it is Important?
- Advantages of Marketing Analytics Certification
- Analytics in Healthcare and the Life Sciences
- What Is a Marketing Analyst? And How to Become One?
- How To Pursue A Career As A Financial Analyst?
- What is Marketing Analytics & Why It Matters?
- Reasons why Financial Analytics is Becoming More Important
- What is Financial Analytics and Why is it Important?
Machine Learning
- Machine Learning — Diagnosing faults in the vehicle
- Kubeflow on Edge Devices: Exploring Opportunities and Constraints
- What is Kubeflow: Role of Istio in Kubeflow
- Introducing the Q Learning : Reinforcement Future of Learning
- What is PyTorch: Revolutionizing Deep Learning
- Reinforcement Learning Algorithms
- Stochastic Gradient Descent: A Comprehensive Guide
- What is Data Drift? : Techniques and How does it works
- What is Concept Drift : Examples and Challenges
- A Comprehensive Guide to Data Drift, Model Drift, and Feature Drift
Internet Of Things
- Why is IoT Dangerous?
- What is the Vulnerability of IoT?
- What is the Future of IoT in India?
- What after IoT?
- What are the Examples of IoT Devices?
- What are the Disadvantages and Limitations of IOT
- What is an IoT Attack?
- How Secure are IoT Devices?
- How Do I Protect my IoT Devices?
- Can IoT Devices be Hacked?
Interview Questions
- Matrices Interview questions and Answers
- Matrices and Calculus Interview questions and Answers
- Numbers Interview questions and Answers
- Odd Man Out Interview questions and Answers
- Odd oneout Interview questions and Answers
- Python Interview questions and Answers
- Python Data types Interview questions and Answers
- Best Python libraries Interview questions and Answers
- Python Loops Interview questions and Answers
- Python Strings Interview questions and Answers
Big Data & Analytics
- The Power of Views: A Comprehensive Way to Power BI's Data Visualization, Business Intelligence, and
- What is Anomaly Detection? Types, Models and Examples
- The Journey to Becoming a Data Analyst: A Step-by-Step Guide
- Data Analytics in the Digital Era: The Future of Work and Career Opportunities
- The Ethical Dilemma: Exploring the Implications of Data Analytics
- Data Analytics Case Studies: Real-World Examples of Business Insights and Success
- Unveiling Hidden Opportunities: Leveraging Data Analytics for Business Growth
- Unleashing the Power: Exploring the Best Data Analytics Tools for Unraveling Insights
- The Future of Data Analytics : Unveiling Tomorrow
- Step by Step Starts Your Data Analytics Journey
Robotic Process Automation
Agile and Scrum Methodology
Industrial Revolution IR4.0
Interview Questions on Data Science
- Logical Expressions Interview Questions and Answers
- Text Mining Interview Questions and Answers
- Ensemble Modeling Interview Questions and Answers
- Lasso & Ridge Regression Interview Questions & Answers in 2024
- Forecasting Time Series Interview Questions & Answers
- Multiple Linear Regression Interview Questions & Answers
- Hierarchical Clustering Interview Questions & Answers
- CRISP-DM Interview Questions & Answers
- Moments of Business Decision
- Business Understanding
Quality Management
Machine Learning
MLOps
- Get to Know Everything About MLOps: What It Is, Why It Matters, and How to Implement It.
- How to become an MLOps Engineer?
- What is MLOps?
- What Differs Between MLOps Engineers & DevOps?
- Get To Know The Difference Between MLOps vs Data Engineering Here
- KNN Classifier
- Pitfalls on only data driven ML approaches
- ML Ops
- How does Zomato make use of Machine learning?
- India will become a semiconductor hub soon!!!!
IT Companies
- Top 25 IT Companies in Myanmar
- Top 20 IT Companies in Cambodia
- Top 11 IT Companies in Brunei
- Top 25 IT Companies in Laos
- Top 7 IT Companies in Faridabad
- Top 9 IT Companies in Guntur
- Top 9 IT Companies in Chandigarh
- Top 8 IT Companies in Mysore
- Top 8 IT Companies in Trichy
- Top 3 IT Companies in Hoodi
Medical Supplier
Health care and safety
Construction companies
Food Industry
Manufacturing companies
BPO Companies
Engineering companies
Semiconductors Companies
Banking companies
Electrical Engineering Companies
Research and development companies
Logistics Companies
F&B companies
Interview Questions on Data Engineering
- Top 50+ ETL Interview Questions For Data Engineering
- Top 35 Data Pipeline Interview Questions
- Top 10 Data Warehouse Interview Questions
- Top 70 Data Transformation Interview Questions
- Top 35 Data Lake Interview Questions and Answers
- Top 35 Apache Kafka Interview Questions
- Top 35 Apache Airflow Interview Questions
- Top 35 Data Source Interview Questions
- Top 35 Data Architect Interview Questions
- Top 35 Data Pipeline Interview Questions and Answers
Generative AI
- Generative AI Interview Questions
- Introducing Rockset Vector Database
- A Step-by-Step DeepLake Vector Database Tutorial
- Qdrant Vector Database
- Redis Vector Database Tutorial Step by Step Guide
- Faiss Vector Database Tutorial Step by Step Guide
- Vald Vector Database
- Unleashing the Power of Elasticsearch Vector Database: A Comprehensive Exploration
- Revolutionizing AI Applications with Pinecone: Unleashing the Power of Vector Databases
- Generated Knowledge
Home / Blog / Data Science Digital Book / Recommender Systems
Recommender Systems
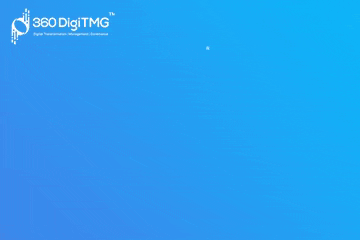
Meet the Author : Mr. Bharani Kumar
Bharani Kumar Depuru is a well known IT personality from Hyderabad. He is the Founder and Director of Innodatatics Pvt Ltd and 360DigiTMG. Bharani Kumar is an IIT and ISB alumni with more than 18+ years of experience, he held prominent positions in the IT elites like HSBC, ITC Infotech, Infosys, and Deloitte. He is a prevalent IT consultant specializing in Industrial Revolution 4.0 implementation, Data Analytics practice setup, Artificial Intelligence, Big Data Analytics, Industrial IoT, Business Intelligence and Business Management. Bharani Kumar is also the chief trainer at 360DigiTMG with more than Ten years of experience and has been making the IT transition journey easy for his students. 360DigiTMG is at the forefront of delivering quality education, thereby bridging the gap between academia and industry.
Recommender Systems is also called Collaborative Filtering.
'Users' are typically the rows in the data utilised for the analysis, and 'Items' will be the columns. (from retail ecommerce context)
Whether a user has
purchased or not
Whether user has rated
the product or not
How many products each
user has purchased?
What is the rating
provided by the user?
Click here to learn Data Science in Hyderabad
The values, such as ratings columns, are occasionally split by frequency. The quantity of buyers or raters is referred to as the frequency. The ratings are normalised throughout this procedure.
applied often on e-commerce sites. To create personalised tactics to propose products with a high likelihood of being purchased, customers' purchasing behaviours are examined.
- What is the item most likely to be purchased?
- Can we identify and make suggestions/recommendations upfront?
It is necessary to respond to these two basic questions. Recommendations in turn assist the user develop confidence in themselves and brand loyalty.
Types of Recommendation Strategies
Learn the core concepts of Data Science Course video on YouTube:
The most often used method is collaborative filtering, which relies on user similarity metrics.
Similarity Measures:
Cos(A,B) = A•B / |A|*|B|
Corr(AB) = Covariance (A,B) / Stdev (A) * Stdev (B)
Euclidean distance
Manhattan distance, etc.
What to Recommend:
List the products that the individual is MOST LIKELY to buy from the list of products that customers with comparable buying patterns have already purchased.
Sorting the list of items can be based on:
- How many similar customers purchased it
- Rated by most
- Highest rated, etc.
Disadvantages:
Costly memory and lazy learning
Compute is relatively costly - n computations for similarities
Alternative Approaches
Making strategic decisions in comparison to suggestions with "Better accuracy and offline" vs. "Slightly lower accuracy and online"
Recommendations vs Association Rules
Challenge with Rating Matrix-Based Recommendation:
The number of vacant cells in rating matrices makes them large and sparse.
The sparse rating matrix is handled using the SVD algorithm.
Data Science Placement Success Story
Data Science Training Institutes in Other Locations
Agra, Ahmedabad, Amritsar, Anand, Anantapur, Bangalore, Bhopal, Bhubaneswar, Chengalpattu, Chennai, Cochin, Dehradun, Malaysia, Dombivli, Durgapur, Ernakulam, Erode, Gandhinagar, Ghaziabad, Gorakhpur, Gwalior, Hebbal, Hyderabad, Jabalpur, Jalandhar, Jammu, Jamshedpur, Jodhpur, Khammam, Kolhapur, Kothrud, Ludhiana, Madurai, Meerut, Mohali, Moradabad, Noida, Pimpri, Pondicherry, Pune, Rajkot, Ranchi, Rohtak, Roorkee, Rourkela, Shimla, Shimoga, Siliguri, Srinagar, Thane, Thiruvananthapuram, Tiruchchirappalli, Trichur, Udaipur, Yelahanka, Andhra Pradesh, Anna Nagar, Bhilai, Borivali, Calicut, Chandigarh, Chromepet, Coimbatore, Dilsukhnagar, ECIL, Faridabad, Greater Warangal, Guduvanchery, Guntur, Gurgaon, Guwahati, Hoodi, Indore, Jaipur, Kalaburagi, Kanpur, Kharadi, Kochi, Kolkata, Kompally, Lucknow, Mangalore, Mumbai, Mysore, Nagpur, Nashik, Navi Mumbai, Patna, Porur, Raipur, Salem, Surat, Thoraipakkam, Trichy, Uppal, Vadodara, Varanasi, Vijayawada, Vizag, Tirunelveli, Aurangabad
Navigate to Address
360DigiTMG - Data Science, IR 4.0, AI, Machine Learning Training in Malaysia
Level 16, 1 Sentral, Jalan Stesen Sentral 5, Kuala Lumpur Sentral, 50470 Kuala Lumpur, Wilayah Persekutuan Kuala Lumpur, Malaysia
+60 19-383 1378