Sent Successfully.
Home / Blog / Data Science / 7 Crucial Proved Key Challenges To Successful And Proven Data Science Projects
7 Crucial Proved Key Challenges To Successful And Proven Data Science Projects
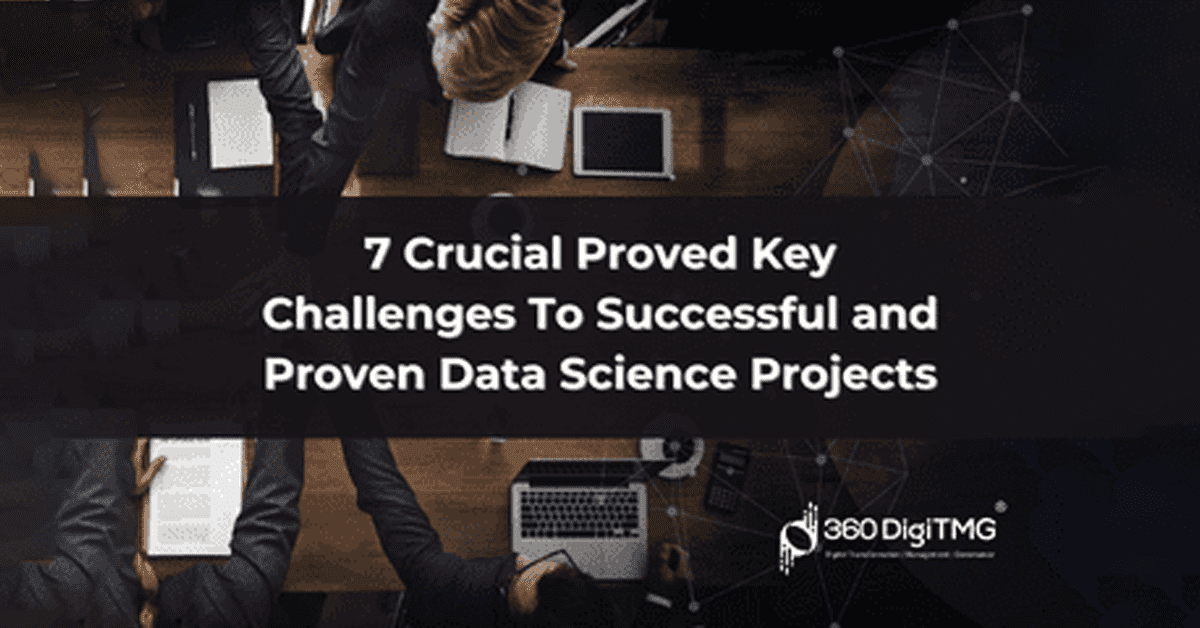
Table of Content
- The complexity of data quality management
- Large-scale data management
- How can we measure the worth of data science projects?
- Uncertainty about the business problem
- The challenging job of transforming big data into useful insights
- Improper management and structure of the data team
- Challenges in finding skilled data scientists for data science projects
Any organisation looking to improve and automate decision-making should consider the immense potential of data science. Decision-makers must instead depend on simple heuristics, guesses, or intuition because the majority of data initiatives don't provide much or any economic value. Similar to this, converting data science proof of concepts into organizational-wide solutions requires a lot of work.
To get employment in data science, however, early career workers need more than just a strong theoretical foundation. Employing managers today are seeking data scientists with real-world experience who have completed projects that solve current concerns. Before you even get your first job, you need to have "experience" proving you can provide them.
Whether you are a total novice or an expert, a logical strategy will assure the success of your activities. In order to successfully complete your data science projects, you can utilise the seven difficulties described in this article.
Become a Data Scientist with 360DigiTMG Data Science course in Hyderabad Get trained by the alumni from IIT, IIM, and ISB.
Learn the core concepts of Data Science Course video on Youtube:
What is a data science project?
You may use a data science project to put your knowledge into action. You may put your understanding of data collection, cleaning, analysis, visualisation, programming, machine learning, and other relevant areas to use in a typical project. You can use your abilities to address problems in the real world. Once finished, you may add this to your portfolio to show potential employers your skills.
7 Key challenges to successful data science projects:
You can maintain a firm grasp on Data Science trends & technologies thanks to your real-time experience on Live Data Science Projects. So start working on current Data Science projects, and you'll soon see how useful they will be for your quick, professional advancement. Now let's examine in detail the challenges to successful data science projects.
1. The complexity of data quality management:
Data from numerous sources: Since the data you must analyse comes from many sources and in many different formats, data integration will unavoidably provide a barrier. For instance, e-commerce companies need to look at data from social media, website logs, call centres, and competing websites' website "scans".
Erroneous data:There is no denying that proper information isn't always available. In general, it's not that significant. That does not, however, imply that you should not exercise any control over how reliable your data is. It might also be duplicated, inconsistent, and include false information.
Comparison of data with the only reliable source of information (such as matching different address spellings to spellings found in the postal system database). Compare and combine records that are connected to the same entity.
Are you looking to become a Data Scientist? Go through 360DigiTMG's PG Diploma in Data Science and Artificial Intelligence!.
2. Large-scale data management:
As we've already mentioned, the amount of data available grows every day. The quantity of data stored in the world's IT systems is doubling every two years, according to the IDC Digital Universe report.
It should not come as a surprise that handling these massive volumes of data offers serious challenges for organisations.
Making critical business choices promptly and effectively necessitates the implementation of a strong infrastructure that can process data more rapidly and offer real-time insights. Businesses are utilising big data platforms more often to handle data storage, administration, cleaning, and analytics in order to extract the insights that their organisations need, when they need them, to cope with the challenge of handling enormous data amounts.
Want to learn more about data science? Enroll in the Best Data Science courses in Chennai to do so.
3. How can we measure the worth of data science projects?
If you look at it from a business standpoint, they often view investments as either introducing cost reductions or potentially increasing future cash flow in terms of revenue. Projects involving data science occasionally directly relate to these two factors and have an immediate effect. But in other circumstances, you might be unable to explain how your solution generated a certain sum of money.
Revenue is simple to measure. However, you may find it challenging to position many of the items you build in terms of revenue. In that situation, you must set up and design a route to the influence that you intend to have. Of course, that implies that you need to market what you're doing.
To communicate the true purpose of what is being built, we must first select high-value problems.
Impact versus measurability is the second point. We must find a means to evaluate the impact. We should consider elements like revenue, cost savings, a speedier time to market, or improving the users' quality of life.
.png)
4. Uncertainty about the business problem:
One should initially do study on the business problem for which data science solutions are desired. It is less beneficial to choose the mechanical approach of finding datasets and performing data analysis before deciding what business problem to address. If you want to utilise data science to make informed decisions, this is really useless. Furthermore, even if you have a specific target in mind, your efforts won't be successful if your expectations for how data science will be applied do not match those goals.
Choosing which use case to solve may be done successfully by developing a great workflow approach. construct a procedure by working with all the departments to construct a checklist that makes it easier to identify problems. This facilitates identifying a business issue in a multidisciplinary context.
Earn yourself a promising career in data science by enrolling in the Data Science Classes in Pune offered by 360DigiTMG.
5. The challenging job of transforming big data into useful insights:
Based only on historical data regarding customer behavior, the super-cool big data analytics examines what product combinations customers purchase (for example, a needle and thread). For example, a specific soccer player posts his new style on Instagram, and two defining elements of it are white Nike sneakers and a beige hat. So naturally, people want to appear like him because he looks fantastic in them. So they run out and get similar headgear and a pair of sneakers. But you sell sneakers in your store. As a result, you lose revenue and perhaps some loyal clients.
Because your big data technology doesn't evaluate data from social networks or rival websites, you failed to have the necessary items in stock. While your rival's big data does, among other things, track social media trends in almost real time. Additionally, their store sells both goods and grants a 15% discount if you buy them both.
The objective is to establish a suitable system of variables and data sources whose analysis will provide critical insights and ensure that nothing is beyond bounds. Even though it could be challenging to collect and analyze external data, such a system should frequently involve external sources.
6. Improper management and structure of the data team
Despite the ongoing discussion about the best ways to organize and lead data teams, few businesses have established the ideal conditions for their data scientists to flourish and advance their data science projects. Data science teams frequently work in compartments like the center of excellence or research and development divisions. Teams dedicated to data science may be separated from business teams and buried deep within IT architecture.
One frequently makes data science teams for data science projects of doctoral-level scientists without the assistance of data and software engineers, led by a lead data scientist who prefers to work alone, has no interpersonal skills, or both. Instead, team leadership and structure should adopt an operating philosophy that puts the client's needs first.
Challenges in finding skilled data scientists for data science projects
Finding a good data team with in-depth knowledge and subject experience is usually difficult for businesses. The business applications of data science as well as the methodologies used in ML and AI must be thoroughly understood by specialists. When an organisation is able to utilise their data to tell their business story, a data science project is viable. For this reason, problem-solving skills are just as important for analysts and scientists as the capacity to convey stories using data.
Because different teams have different workflows and priorities, it is impossible to emphasise how crucial consensus is among all teams. Professionals should be able to adequately explain technological challenges to business owners. However, it might be difficult to find such a team. An ideal substitute is a corporation that uses data science. A data science firm is a great option to get in touch with since they have the required technical expertise, are aware of the project's commercial ramifications, and are willing to commit to it.
Wrapping Up:
In conclusion, you can take on more advanced projects once you feel confident. Get hold of these data science project ideas if you want to sharpen your data science skills. Build your data science project today to test all the knowledge you've learned from our guide to data science project ideas!
Data Science Placement Success Story
Data Science Training Institutes in Other Locations
Agra, Ahmedabad, Amritsar, Anand, Anantapur, Bangalore, Bhopal, Bhubaneswar, Chengalpattu, Chennai, Cochin, Dehradun, Malaysia, Dombivli, Durgapur, Ernakulam, Erode, Gandhinagar, Ghaziabad, Gorakhpur, Gwalior, Hebbal, Hyderabad, Jabalpur, Jalandhar, Jammu, Jamshedpur, Jodhpur, Khammam, Kolhapur, Kothrud, Ludhiana, Madurai, Meerut, Mohali, Moradabad, Noida, Pimpri, Pondicherry, Pune, Rajkot, Ranchi, Rohtak, Roorkee, Rourkela, Shimla, Shimoga, Siliguri, Srinagar, Thane, Thiruvananthapuram, Tiruchchirappalli, Trichur, Udaipur, Yelahanka, Andhra Pradesh, Anna Nagar, Bhilai, Borivali, Calicut, Chandigarh, Chromepet, Coimbatore, Dilsukhnagar, ECIL, Faridabad, Greater Warangal, Guduvanchery, Guntur, Gurgaon, Guwahati, Hoodi, Indore, Jaipur, Kalaburagi, Kanpur, Kharadi, Kochi, Kolkata, Kompally, Lucknow, Mangalore, Mumbai, Mysore, Nagpur, Nashik, Navi Mumbai, Patna, Porur, Raipur, Salem, Surat, Thoraipakkam, Trichy, Uppal, Vadodara, Varanasi, Vijayawada, Visakhapatnam, Tirunelveli, Aurangabad
Data Analyst Courses in Other Locations
ECIL, Jaipur, Pune, Gurgaon, Salem, Surat, Agra, Ahmedabad, Amritsar, Anand, Anantapur, Andhra Pradesh, Anna Nagar, Aurangabad, Bhilai, Bhopal, Bhubaneswar, Borivali, Calicut, Cochin, Chengalpattu , Dehradun, Dombivli, Durgapur, Ernakulam, Erode, Gandhinagar, Ghaziabad, Gorakhpur, Guduvanchery, Gwalior, Hebbal, Hoodi , Indore, Jabalpur, Jaipur, Jalandhar, Jammu, Jamshedpur, Jodhpur, Kanpur, Khammam, Kochi, Kolhapur, Kolkata, Kothrud, Ludhiana, Madurai, Mangalore, Meerut, Mohali, Moradabad, Pimpri, Pondicherry, Porur, Rajkot, Ranchi, Rohtak, Roorkee, Rourkela, Shimla, Shimoga, Siliguri, Srinagar, Thoraipakkam , Tiruchirappalli, Tirunelveli, Trichur, Trichy, Udaipur, Vijayawada, Vizag, Warangal, Chennai, Coimbatore, Delhi, Dilsukhnagar, Hyderabad, Kalyan, Nagpur, Noida, Thane, Thiruvananthapuram, Uppal, Kompally, Bangalore, Chandigarh, Chromepet, Faridabad, Guntur, Guwahati, Kharadi, Lucknow, Mumbai, Mysore, Nashik, Navi Mumbai, Patna, Pune, Raipur, Vadodara, Varanasi, Yelahanka
Navigate to Address
360DigiTMG - Data Science Course, Data Scientist Course Training in Chennai
D.No: C1, No.3, 3rd Floor, State Highway 49A, 330, Rajiv Gandhi Salai, NJK Avenue, Thoraipakkam, Tamil Nadu 600097
1800-212-654-321