Sent Successfully.
Home / Blog / Data Science / What is Data Mining: A Comprehensive Overview
What is Data Mining: A Comprehensive Overview
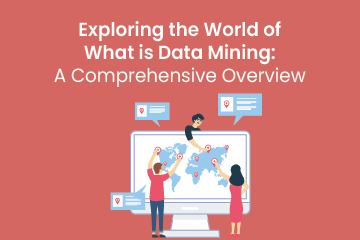
Table of Content
- What is Data Mining?
- Techniques and Methods Used in Data Mining
- Applications of Data Mining
- Data Mining Techniques and Algorithms
- Overview of Ethical Considerations in Data Mining
- Challenges in Data Cleaning, Missing Data, and Overfitting
- Explanation of how Data Mining will Continue to Evolve and Shape the Future
- Conclusion
In today's world, data is everywhere. From business transactions to social media activity, we generate massive amounts of data every day. But all this data is useless unless we can extract valuable insights from it. This is where data mining comes in. Data mining is nothing but the process of discovering patterns, correlations, and insights in large data sets to extract meaningful information. It's a powerful tool that is used in a variety of industries, such as like from finance to healthcare to marketing. In this blog post, we'll explore the world of data mining and provide a comprehensive overview of what it is, how it works, and its applications in various industries. We'll also discuss what are the challenges and ethical considerations in data mining, as well as the future of this rapidly evolving field. By the end of this post, you'll have a better understanding of the data mining and its potential to transform the way we extract insights from data.
What is Data Mining?
It is a process of discovering patterns, correlations, and insights in large data sets to extract meaningful information. It's a powerful tool that is used in various industries, including finance, healthcare, marketing, and more. By applying various techniques and methods, data mining helps to uncover hidden patterns in data and turn raw data into actionable intelligence. In this section, we'll delve deeper into the definition of data mining and explore the different techniques and methods used in the process.
Learn the core concepts of Data Science Course video on YouTube:
Techniques and Methods Used in Data Mining
There are several techniques and methods used in data mining, including:
• Association Rule Learning: It is discovering relationships between variables in large data sets.
• Clustering: It is about grouping similar data points together to identify the patterns and relationships.
• Classification: Categorizing data into predefined classes or groups based on certain characteristics.
• Regression Analysis: It is about identifying the relationship between two variables, that are a dependent variable and one or more independent variables.
• Neural Networks: Modelling the way the human brain works to analyze data and identify patterns.
Overall, data mining is a valuable tool that helps businesses and organizations to make informed decisions based on large sets of data. By uncovering hidden patterns and relationships within the data, data mining can help to improve business operations, increase efficiency, and drive growth.
Being a Data Scientist is just a step away. Check out the Data Science Course with Placement at 360DigiTMG and get certified today.
Applications of Data Mining:
In today's data-driven world, businesses and organizations generate and collect vast amounts of the data every day. But the value of this data lies in its ability to provide insights and improve decision-making. This is where data mining comes in. Data mining is a powerful tool that enables businesses and organizations to extract the valuable insights from their data by identifying patterns, trends, and relationships that may not be immediately apparent through traditional data analysis methods. In this blog post, we'll explore the various applications of data mining in different industries, including finance, healthcare, retail, and more. We'll also discuss the benefits and challenges of data mining and how it can be used to drive growth and improve operations. By the end of this post, you'll have a better understanding of the diverse applications of data mining and its potential to transform the way businesses and organizations extract value from their data.
Become a Data Scientist with 360DigiTMG best data science institute in Chennai with placement. Get trained by the alumni from IIT, IIM, and ISB.
Examples of how the data mining is used in business, healthcare, and finance:
Examples of Data Mining in Business:
- Retail: Retail companies use data mining to analyze customer behavior and purchase history to identify trends and make informed decisions about product placement and pricing.
- Marketing: Data mining is used in marketing to analyze customer data, segment customers, and personalize marketing campaigns based on customer preferences and behavior.
- Customer Service: Companies use data mining to analyze customer complaints and feedback to identify trends and make improvements to their products or services.
Examples of Data Mining in Healthcare:
- Electronic Health Records: Healthcare providers use data mining to analyze electronic health records to identify patterns and trends in patient data, such as disease risk factors or treatment outcomes.
- Drug Development: Data mining is used in drug development to analyze clinical trial data to identify potential drug targets or side effects.
- Public Health: Public health organizations use data mining to track and analyze disease outbreaks and patterns to develop prevention and treatment strategies.
Examples of Data Mining in Finance:
- Fraud Detection: Financial institutions use data mining to detect fraudulent activities and transactions by analyzing patterns and anomalies in customer behavior and financial transactions.
- Credit Risk Assessment: Banks use data mining to assess credit risk by analyzing customer data to predict the likelihood of loan defaults or delinquencies.
- Investment Analysis: Financial analysts use data mining to analyze market trends and predict investment outcomes.
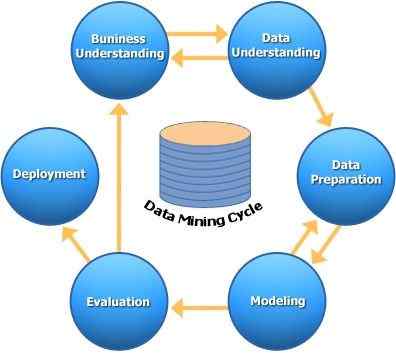
Data Mining Techniques and Algorithms
Data mining techniques and algorithms are used to extract insights and knowledge from large data sets. There are several techniques and algorithms used in data mining, each with its own strengths and limitations. Here are some of the most crucial and common data mining techniques and algorithms:
1. Association Rule Learning: This technique is used to identify patterns and relationships between different variables in a large data set. It involves finding items that are frequently associated with each other and using this information to make predictions or recommendations.
2. Clustering: Clustering is used to group similar data points together based on their characteristics. This technique is useful for identifying patterns and relationships within the data set.
3. Classification: This technique is used to categorize data into predefined classes or groups based on specific characteristics. It is useful for predicting future outcomes or behavior based on past data.
4. Regression Analysis: Regression analysis is used to identify the connection between a dependent variable and one or more independent variables. It is useful for predicting future trends and outcomes based on past data.
5. Decision Trees: Decision trees are a visual representation of a series of decisions and their possible consequences. This algorithm is useful for identifying the most important factors that influence a decision or outcome.
6. Neural Networks: Neural networks are machine learning algorithm that models the way the human brain works to analyze data and identify patterns. They are useful for identifying complex patterns and relationships within a data set.
7. Support Vector Machines: These are a machine learning algorithm that are used for classification and regression analysis. They work by identifying the best boundary between different classes in the data set.
Becoming a Data Scientist is possible now with the 360DigiTMG Data Science Course in Pune with placement program. Enroll today.
Overview of Ethical Considerations in Data Mining
Ethical considerations in data mining related to the use of data in ways that respect privacy, autonomy, and fairness. This means that data mining practices should be transparent, responsible, and respectful of individuals' rights to privacy and confidentiality. It's essential that organizations using data mining techniques take steps to protect the privacy of the individuals whose data is being analyzed and ensure that their methods are fair and unbiased
1. Data privacy: Data mining involves analyzing large datasets, which can include sensitive personal information. It's important to ensure that this data is collected and used in compliance with data protection laws and that individuals' privacy is respected. Organizations should implement appropriate security measures to protect data from unauthorized access and use.
2. Data ownership: In many cases, the data being analyzed in data mining belongs to individuals, not organizations. It's important to obtain informed consent from individuals before using their data and to ensure that individuals understand how their data will be used.
3. Informed consent: Informed consent means that individuals understand how their data will be used and have given their explicit permission for it to be used in that way. Organizations should obtain informed consent from individuals before collecting and using their data in data mining.
4. Fairness: Data mining algorithms can introduce biases, particularly if they are based on historical data that reflects systemic biases. It's important to ensure that data mining algorithms are fair and unbiased and that they do not discriminate against certain individuals or groups.
5. Transparency: Data mining practices should be transparent, meaning that individuals understand how their data is being used and can access information about the data being collected and analyzed. Organizations should provide clear and accessible information about their data mining practices.
Don't delay your career growth, kickstart your career by enrolling in this Data Science Training Institute in Hyderabad with 360DigiTMG Data Scientist Course.
Challenges in Data Cleaning, Missing Data, and Overfitting
Data mining is a valuable tool for extracting insights and knowledge from large data sets, but it comes with its own group of challenges. Three common challenges in data mining are data cleaning, missing data, and overfitting.
1. Data Cleaning: It is the process of identifying and correcting errors, inconsistencies, and discrepancies in a data set. It is an essential step in data mining, as the accuracy and quality of the data will directly impact the accuracy and reliability of the insights gained from the data. However, data cleaning can be a challenging task as it can be time-consuming and requires a thorough understanding of the data and the domain in which it is collected.
2. Missing Data: Missing data is a common problem in data mining, as data sets often have incomplete or missing data. This can occur because of various reasons, such as data collection errors, data entry errors, or data loss during transfer. Handling missing data is a challenging task as it requires careful consideration of the data and the domain in which it is collected. There are various techniques used for handling missing data, such as imputation, where missing data is estimated based on other available data points.
3. Overfitting: It occurs when a model is getting trained too well on the training data, resulting in it being too complex and having poor performance on new data. This is a common problem in data mining, especially when the model is overfitted on a small sample size or biased data. Overfitting can be challenging to identify and can lead to inaccurate insights and predictions. To avoid overfitting, it is very important to use a suitable model selection and validation technique, such as cross-validation, and to ensure that the data used for training and testing is representative of the population being studied.
Explanation of how Data Mining will Continue to Evolve and Shape the Future
Data mining has become a critical tool for organizations in many industries, and its importance is only set to increase as technology continues to advance. Here are some ways that data mining is likely to evolve and shape the future
1. Increasingly sophisticated algorithms: As data sets become larger and more complex, data mining algorithms will need to become more sophisticated to keep up. Advances in machine learning and artificial intelligence will help data mining algorithms learn from patterns in data and improve their accuracy.
2. More data sources: As more and more data is generated from sources such as IoT devices and social media, data mining algorithms will need to adapt to handle this new data. This will require new techniques and algorithms to analyze and extract insights from a wider range of data sources.
3. Real-time processing: Real-time data processing is becoming increasingly important, particularly in industries such as finance and healthcare where decisions need to be made quickly. Data mining algorithms will need to be able to process data in real-time to provide insights that can be acted upon immediately.
4. Improved data privacy and security: As more data is collected and analyzed, data privacy and security will become even more important. Data mining algorithms will need to be designed to ensure that sensitive data is protected and that privacy laws are complied with.
5. Integration with other technologies: Data mining is likely to become more integrated with other technologies such as artificial intelligence, machine learning, and natural language processing. This will enable organizations to extract insights from data more efficiently and accurately.
Want to learn more about data science? Enroll in this Data Science Course Training in Bangalore to do so.
Conclusion
In the bottom line, data mining is a powerful tool that provides valuable insights and benefits for organizations in various industries. However, it also raises important ethical considerations related to privacy, fairness, and autonomy. It's important for the organizations to carefully consider these ethical considerations and develop responsible and transparent data mining practices.
Data Science Placement Success Story
Data Science Training Institutes in Other Locations
Agra, Ahmedabad, Amritsar, Anand, Anantapur, Bangalore, Bhopal, Bhubaneswar, Chengalpattu, Chennai, Cochin, Dehradun, Malaysia, Dombivli, Durgapur, Ernakulam, Erode, Gandhinagar, Ghaziabad, Gorakhpur, Gwalior, Hebbal, Hyderabad, Jabalpur, Jalandhar, Jammu, Jamshedpur, Jodhpur, Khammam, Kolhapur, Kothrud, Ludhiana, Madurai, Meerut, Mohali, Moradabad, Noida, Pimpri, Pondicherry, Pune, Rajkot, Ranchi, Rohtak, Roorkee, Rourkela, Shimla, Shimoga, Siliguri, Srinagar, Thane, Thiruvananthapuram, Tiruchchirappalli, Trichur, Udaipur, Yelahanka, Andhra Pradesh, Anna Nagar, Bhilai, Borivali, Calicut, Chandigarh, Chromepet, Coimbatore, Dilsukhnagar, ECIL, Faridabad, Greater Warangal, Guduvanchery, Guntur, Gurgaon, Guwahati, Hoodi, Indore, Jaipur, Kalaburagi, Kanpur, Kharadi, Kochi, Kolkata, Kompally, Lucknow, Mangalore, Mumbai, Mysore, Nagpur, Nashik, Navi Mumbai, Patna, Porur, Raipur, Salem, Surat, Thoraipakkam, Trichy, Uppal, Vadodara, Varanasi, Vijayawada, Visakhapatnam, Tirunelveli, Aurangabad
Data Analyst Courses in Other Locations
ECIL, Jaipur, Pune, Gurgaon, Salem, Surat, Agra, Ahmedabad, Amritsar, Anand, Anantapur, Andhra Pradesh, Anna Nagar, Aurangabad, Bhilai, Bhopal, Bhubaneswar, Borivali, Calicut, Cochin, Chengalpattu , Dehradun, Dombivli, Durgapur, Ernakulam, Erode, Gandhinagar, Ghaziabad, Gorakhpur, Guduvanchery, Gwalior, Hebbal, Hoodi , Indore, Jabalpur, Jaipur, Jalandhar, Jammu, Jamshedpur, Jodhpur, Kanpur, Khammam, Kochi, Kolhapur, Kolkata, Kothrud, Ludhiana, Madurai, Mangalore, Meerut, Mohali, Moradabad, Pimpri, Pondicherry, Porur, Rajkot, Ranchi, Rohtak, Roorkee, Rourkela, Shimla, Shimoga, Siliguri, Srinagar, Thoraipakkam , Tiruchirappalli, Tirunelveli, Trichur, Trichy, Udaipur, Vijayawada, Vizag, Warangal, Chennai, Coimbatore, Delhi, Dilsukhnagar, Hyderabad, Kalyan, Nagpur, Noida, Thane, Thiruvananthapuram, Uppal, Kompally, Bangalore, Chandigarh, Chromepet, Faridabad, Guntur, Guwahati, Kharadi, Lucknow, Mumbai, Mysore, Nashik, Navi Mumbai, Patna, Pune, Raipur, Vadodara, Varanasi, Yelahanka